汤伟,王杰.基于BI-TCP卷积神经网络的纸机轴承故障诊断方法研究[J].中国造纸学报,2023,38(1):74-81 |
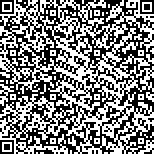 二维码(扫一下试试看!) |
基于BI-TCP卷积神经网络的纸机轴承故障诊断方法研究 |
Fault Diagnosis Method for Paper Machine Bearing Based on BI-TCP Convolutional Neural Network |
投稿时间:2022-09-28 |
DOI:10.11981/j.issn.1000-6842.2023.01.74 |
中文关键词: 纸机轴承 故障诊断 深度学习 双模态输入 特征融合 |
Key Words:bearing fault diagnosis deep learning bimodal input feature fusion |
基金项目:国家自然科学基金项目(62073206)。 |
|
摘要点击次数: 1740 |
全文下载次数: 1099 |
中文摘要: |
针对传统纸机轴承故障诊断模型无法有效提取轴承振动信号深层特征而导致诊断精度难以提高,基于深度学习网络模型参数量过大的问题,提出了一种基于双模态输入和双通道并行卷积神经网络(BI-TCP-CNN)的纸机轴承故障诊断方法。该方法首先在输入数据方面进行创新,将轴承振动信号分别转化为对应的格拉姆角场(GAF)矩阵与欧式距离矩阵,并将二者成对组成的二维矩阵作为网络的输入;其次,利用双通道并行的浅层卷积神经网络提取信号中的不同特征并进行融合,在提高特征完备表达的同时大幅降低了模型的参数量。在凯斯西储大学(CWRU)数据集与实验室搭建的故障诊断实物平台上进行的实物验证结果表明,该方法在CWRU数据集以及实物平台上的故障识别精度均在99.2%以上,可很好地实现对纸机轴承的故障诊断。 |
Abstract: |
Traditional paper machine bearing fault diagnosis models cannot effectively extract the deep characteristics of the signal, hence it is difficult to improve the diagnostic accuracy; moreover, the amount of parameters based on the deep learning network model is too large; therefore, a rolling bearing fault diagnosis method was proposed based on the bimodal input and double-channel parallel convolutional neural network (BI-TCP-CNN) to solve the abovementioned issues. Firstly, the method innovated in the input of the network, converting the original signal into the Gramian Angular Field (GAF) and Euclidean distance matrixes, and a two dimensional matrix composed of the above two matrixes was used as the input of the neural network. Secondly, the BI-TCP-CNN was used to extract different features in the signal and fuse them to improve the complete expression of the features as well as reduce the parameter amount of the model. Finally, the mothod was experimentally verified on the laboratory dataset and fault diagnosis experimental platform of Case Western Reserve University (CWRU). The results show that the accuracies of the obstacle identification of the proposed method in both the CWRU dataset and experimental platform exceeded 99.2%, demonstrating satisfactory realization of the diagnosis of bearing faults. |
查看全文 查看/发表评论 下载PDF阅读器 HTML |