|
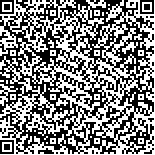 二维码(扫一下试试看!) |
Soft Sensor Modeling of Papermaking Waste Water Treatment Process Using PCA Dimensional Reduction Models |
|
DOI:10.11981/j.issn.1000-6842.2018.04.50 |
Key Words:papermaking waste water treatment processes; principal component analysis; support vector regression; artificial neural network; partial least squares; soft sensor |
Fund Project:制浆造纸工程国家重点实验室开放基金资助项目(201813,201610);南京林业大学高层次人才科研启动基金(163105996);江苏省制浆造纸科学与技术重点实验室开放基金项目(201530)。 |
Author Name | Affiliation | LIU Hong-bin1,2,3,* | 1. Jiangsu Provincial Key Lab of Pulp and Paper Science and Technology, Nanjing Forestry University, Nanjing, Jiangsu Province, 210037 2. State Key Laboratory of Pulp and Paper Engineering, South China University of Technology, Guangzhou, Guangdong Province, 510640 3. Co-Innovation Center of Efficient Processing and Utilization of Forest Resources, Nanjing Forestry University, Nanjing, Jiangsu Province, 210037 | LI Xiang-yu1 | 1. Jiangsu Provincial Key Lab of Pulp and Paper Science and Technology, Nanjing Forestry University, Nanjing, Jiangsu Province, 210037 | YANG Chong1 | 1. Jiangsu Provincial Key Lab of Pulp and Paper Science and Technology, Nanjing Forestry University, Nanjing, Jiangsu Province, 210037 |
|
Hits: 4469 |
Download times: 1182 |
Abstract: |
To deal with the complicated characteristics of papermaking waste water treatment process, two new soft sensors based on dimensional reduction models, where principal component analysis (PCA) was combined with support vector regression (SVR) and artificial neural network (ANN), respectively, were developed for the prediction of the effluent chemical oxygen demand (COD) concentration and the effluent suspended solids (SS) concentration in a papermaking waste water treatment process. Conventional modeling methods including partial least squares (PLS), SVR, and ANN were used for comparison purpose. The results showed that the prediction performance of the PCA-based dimensional reduction models was better than that of the conventional models. Furthermore, PCA-based ANN (PCA-ANN) showed the most accurate prediction results. In terms of the prediction of the effluent COD concentration, the coefficient of determination (R2) and mean square error (MSE) of PCA-ANN were 0.984 and 1.892, respectively, which were optimized by 9.7% and 71.5% in comparison with ANN respectively. Compared with the prediction results of the effluent SS concentration based on ANN, the R2 (0.762) of PCA-ANN was increased by 31.2% and the MSE (0.228) of PCA-ANN was decreased by 58.7%. |
View Full Text View/Add Comment Download reader HTML |