|
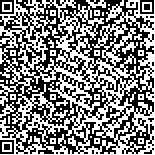 二维码(扫一下试试看!) |
Prediction of Effluent Quality in Wastewater Treatment Systems Using Relevance Vector Machine |
|
DOI: |
Key Words:relevance vector machine; dimensionality reduction; support vector machine; papermaking wastewater treatment process; soft sensor |
Fund Project:制浆造纸工程国家重点实验室开放基金资助项目(201813);南京林业大学高层次人才科研启动基金(GXL029)。 |
Author Name | Affiliation | LIU Hongbin1,2,* | 1. Jiangsu Co-Innovation Center of Efficient Processing and Utilization of Forest Resources,Nanjing Forestry University, Nanjing, Jiangsu Province, 210037 2. State Key Laboratory of Pulp and Paper Engineering, South China University of Technology, Guangzhou, Guangdong Province, 510640 | SONG Liu1 | 1. Jiangsu Co-Innovation Center of Efficient Processing and Utilization of Forest Resources,Nanjing Forestry University, Nanjing, Jiangsu Province, 210037 |
|
Hits: 4082 |
Download times: 1196 |
Abstract: |
Predicting effluent quality precisely is important for papermaking wastewater treatment processes. In this paper, a soft sensor model based on relevance vector machine (RVM) was proposed to predict the effluent quality in a papermaking wastewater treatment plant. The proposed method included two steps. In the first step, partial least squares (PLS) method was used to extract the latent variables of the papermaking process data, which could solve the problems of collinearity and high dimensionality in the process variables. A RVM model using the extracted latent variables was developed in the second step. The results showed that the prediction performance of the PLS-RVM was better than that of the RVM for the prediction of the effluent quality in a papermaking wastewater treatment plant. With regard to the prediction of effluent suspension solids (SS), the root mean square error (RMSE) was decreased by 7.76% and the determination coefficient (R2) was increased by 12.32%; however, the prediction results were not improved significantly for the prediction of effluent chemical oxygen demand (COD). In addition, the prediction performance of the PLS-RVM was better than that of the PLS-LSSVM model for both the effluent SS and the effluent COD. In terms of the prediction of the effluent SS, the RMSE was decreased by 9.16% and the R2 was increased by 15.29%. In terms of the prediction of the effluent COD, the RMSE was decreased by 9.29% and the R2 was increased by 18.34%. |
View Full Text View/Add Comment Download reader HTML |