|
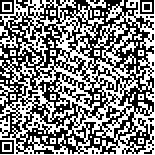 二维码(扫一下试试看!) |
Fault Diagnosis Method for Paper Machine Bearing Based on BI-TCP Convolutional Neural Network |
Received:September 28, 2022 |
DOI:10.11981/j.issn.1000-6842.2023.01.74 |
Key Words:bearing;fault diagnosis;deep learning;bimodal input;feature fusion |
Fund Project:国家自然科学基金项目(62073206)。 |
Author Name | Affiliation | Postcode | TANG Wei* | School of Electrical and Control Engineering, Shaanxi University of Science and Technology, Xi’an, Shaanxi Province, 710021 | 710021 | WANG Jie | School of Electrical and Control Engineering, Shaanxi University of Science and Technology, Xi’an, Shaanxi Province, 710021 | 710021 |
|
Hits: 2028 |
Download times: 1212 |
Abstract: |
Traditional paper machine bearing fault diagnosis models cannot effectively extract the deep characteristics of the signal, hence it is difficult to improve the diagnostic accuracy; moreover, the amount of parameters based on the deep learning network model is too large; therefore, a rolling bearing fault diagnosis method was proposed based on the bimodal input and double-channel parallel convolutional neural network (BI-TCP-CNN) to solve the abovementioned issues. Firstly, the method innovated in the input of the network, converting the original signal into the Gramian Angular Field (GAF) and Euclidean distance matrixes, and a two dimensional matrix composed of the above two matrixes was used as the input of the neural network. Secondly, the BI-TCP-CNN was used to extract different features in the signal and fuse them to improve the complete expression of the features as well as reduce the parameter amount of the model. Finally, the mothod was experimentally verified on the laboratory dataset and fault diagnosis experimental platform of Case Western Reserve University (CWRU). The results show that the accuracies of the obstacle identification of the proposed method in both the CWRU dataset and experimental platform exceeded 99.2%, demonstrating satisfactory realization of the diagnosis of bearing faults. |
View Full Text View/Add Comment Download reader HTML |